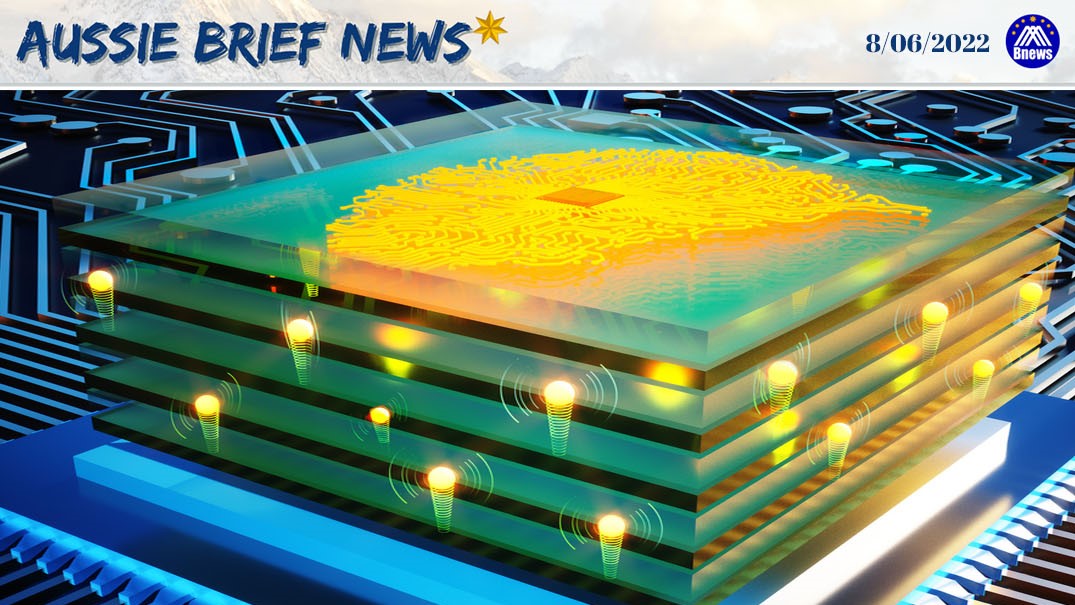
An interdisciplinary team at MIT has recently proposed a new field of artificial intelligence for analog deep learning, involving the use of inorganic phosphosulfate glass (PSG), an electrolyte material, to create a super-fast protonic programmable resistor that can accelerte deep learning in a more energy-efficient mode. It will help scientists develop deep learning models for practical applications more quickly, such as self-driving cars, medical image analysis, and more.
Analog deep learning has two advantages over its digital counterpart in terms of faster speed and greater energy efficiency. First, computation is performed in memory, saving time spent transferring data back and forth between memory and the processor. Second, analog processors operate in parallel, a faster mode than serial ones. Learning in the human brain occurs through the enhancement and weakening of connections between neurons, and deep neural networks have long adopted this strategy to use train algorithms to program the network weights.
The key component of the analog deep learning proposed by the team is a protonic programmable resistor, which can use to arrange an array at the nanoscale, similar to a transistor, the core device of a digital processor. The researchers can repeat arrays of programmable resistors in complex layers to create a network of analog artificial neurons and synapses, which perform computations like digital neural networks. Such networks can be trained to perform complex AI tasks, including image recognition and natural language processing.
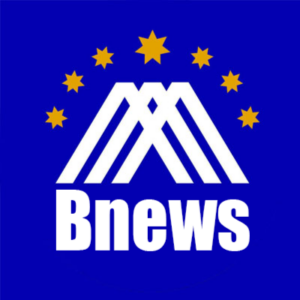
Aussie Brief News
Go to First Page and Get the Latest News.